Earlier this year, I wrote briefly about AI as one of the narratives that would drive the market in 2024. Today’s article aims to dig into some of the issues surrounding that topic.
So far, the AI narrative has helped drive outsized returns for a narrow group of AI-focused infrastructure (semiconductor) companies. The market has now begun to look at the second- and third-derivatives of the AI story, which has invited new groups of unlikely winners to the party including utilities and energy infrastructure providers. That’s occurred as investors have grappled with these facts:
- AI requires significant amounts of energy to run, water to cool, and data to train. All of these things are scarce resources.
- Most standalone AI companies are not profitable yet. Reaching profitability will require consumers to change behavior, something not easily accomplished.
- AI laws and regulations lag behind AI innovation. Regulations and laws will eventually catch up and could impact profitability and applications of AI.
While all of these issues are important, I would like to focus primarily on the energy issue, as I believe it’s currently the most significant of the three. AI is a hungry beast. Like the hungry plant in Little Shop of Horrors, it’s demanding to be fed … but how to feed it is by no means straightforward.
Power hungry
Current estimates indicate that there are about 8,000 data centers in the world, with the bulk of those here in the US. A single data center can hold thousands of servers; these servers are where the AI “lives.” Each server might hold multiple processing units (chips) coupled with a variety of other high-tech components.
Let’s zoom in even more. Each processing unit can hold billions of tiny transistors, each of which is smaller than the thickness of a human hair. (To fully understand the level of complexity here, I highly recommend this amazing visual journey about microchips from the Financial Times.)
As you can imagine, designing and producing something that small and that advanced is extremely expensive and takes significant research and development. That’s why the work lies with just a handful of advanced semiconductor companies. Nvidia is one of the biggest names in AI right now because it designs and sells some of the most advanced semiconductor chips that operate as the brains of the AI operation.
But just like our human brains, a GPU can’t live on its own. A whole host of other components need to support it, and all of these things need a lot of energy to work.
The energy demand is where AI runs into trouble. Energy demand in the US has been flat for years, but some recent energy growth projections I have seen now show roughly 2.5% growth, mostly from increasing computing power usage. (See the expanding maroon bars in the accompanying chart.)
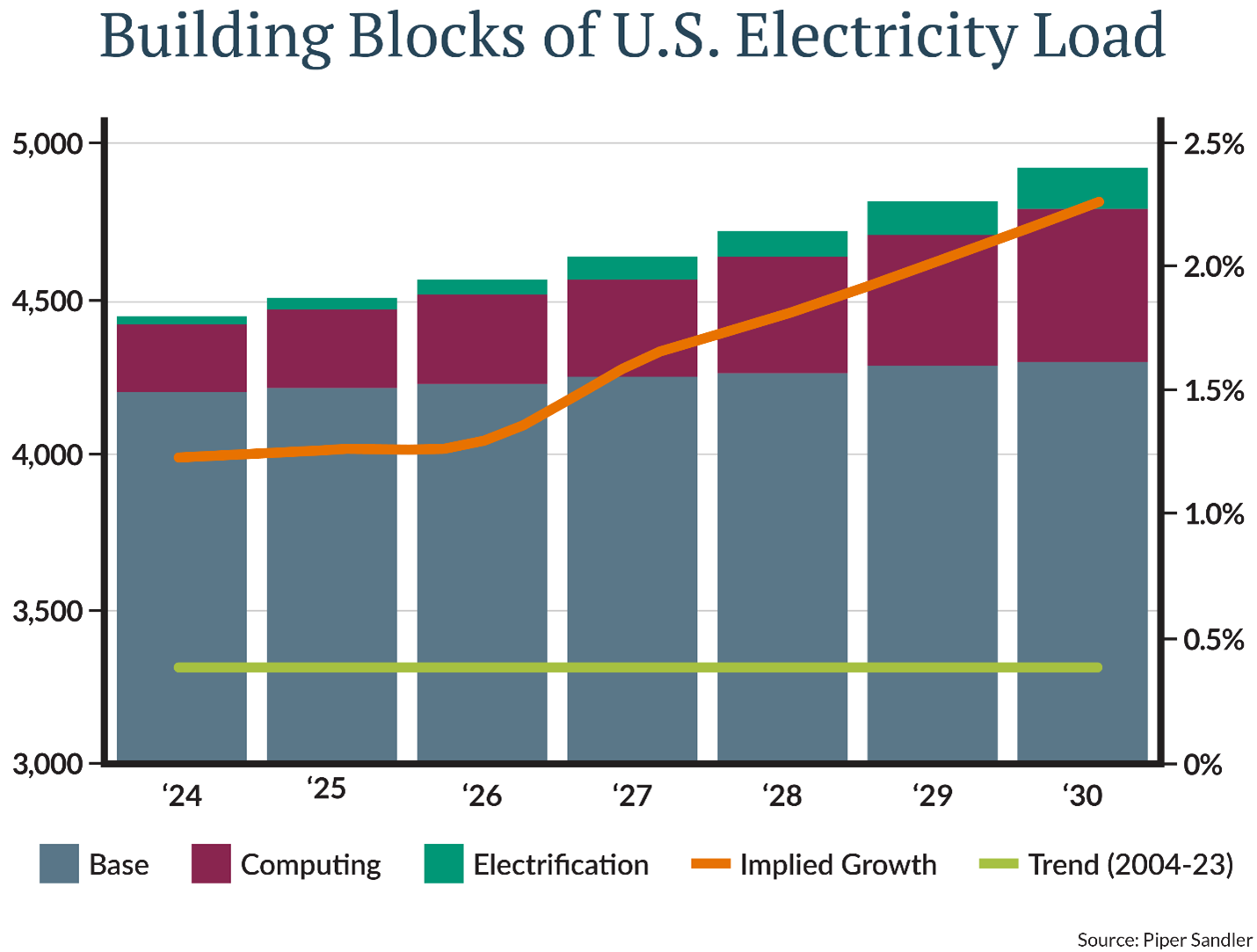
A recent U.S. Department of Energy paper titled “AI for Energy, Opportunities for a Modern Grid and Clean Energy Economy” helps explain the problem of meeting that increased demand:
“The electrical grid of the United States is among the most complex machines on earth. It consists of tens of thousands of power generators delivering electricity across more than 600,000 circuit miles of transmission lines, 70,000 substations, 5.5 million miles of distribution lines, and 180 million power poles. This system evolved organically over a century of piecemeal additions, and now operates at the heart of America’s $28 trillion economy (GDP, Q4 2023). While the grid is generally highly reliable today, this infrastructure is becoming old and overburdened, and outages already cost American businesses $150 billion annually. Several coinciding trends – including electrification, renewable power growth, growing demand, and the rising threat of wildfires and other climate-change-related weather events – are now putting new pressures on a grid that was built on unidirectional energy flows and with very little performance information from sensors.”
My simple interpretation: Our new, futuristic, power-hungry tech is relying on a 100-year-old electrical grid that is held together with duct tape and twine.
Power sources, water supply … and data
The IEA estimates that by 2026 data centers could consume as much energy as the entire country of Japan. Similarly, Microsoft, one of the biggest proponents of AI, has seen its energy usage more than double since 2020. Per my own calculations, Microsoft’s energy consumption in 2023 is now roughly equivalent to the energy used by all the households in Wisconsin combined … plus an additional 136,000 households!
This concern over AI’s power consumption has caused places like the Netherlands, and Ireland to put moratoriums on new data center construction. I suppose you could say they have prioritized light switches and air conditioning over AI applications.
Some U.S. cities may soon be following suit. Even AI’s golden boy, Sam Altman (CEO of OpenAI), has acknowledged that we need a breakthrough in energy to power AI to its full potential. This is why you are seeing more and more talk about nuclear—and why people like Altman are investing in nuclear-focused companies.
Energy is not the only thing the hungry AI beast needs to survive. AI also needs water for cooling. The servers (where the AI lives) generate heat and need to be cooled down so they don’t overheat and shut down. Data centers in Iowa have been in the news recently for their water consumption because much of Iowa is currently suffering from a drought. A recent story highlighted the fact that a data center in Altoona, Iowa is consuming about 20% of the water that the entire city uses. We can look at Microsoft’s annual sustainability publications again for some real numbers. In 2023, Microsoft consumed 7,844 Megaliters of water, an 87% increase in water consumption from 2020, and roughly equivalent to 3,138 Olympic pools worth of water.
Lastly, much like a kid going to school, AI needs data so it can learn. The GIGO (Garbage in Garbage out) principle certainly applies to AI; high-quality data is important. Some estimates indicate that “high-quality language stock” used for training Large Language Models (think ChatGPT and equivalents) will be exhausted this year.
Will a lack of quality data hinder the growth potential of AI? Those of you who grew up thinking about SkyNet (from the “Terminator” movies), as I did, may think stunting the hungry beast is actually the best outcome.